The healthcare industry has shifted paradigms in recent years due to the rapid integration of digital technologies, such as artificial intelligence (AI). While patients have long embraced the internet and informal online sources to understand their conditions, a new trend is emerging: healthcare professionals’ increasing reliance on generative AI.
Unlike traditional AI models focused on data analysis and interpretation, generative AI systems can create new content. This remarkable capability, coupled with user-friendly interfaces, has sparked a surge in the use of generative AI across various industries, including healthcare. As healthcare providers explore the potential of this technology, it is crucial to find a balance between harnessing its benefits and minimizing unintended consequences.
While using generative AI solutions in healthcare has shown promising results, it is not a cure-all. Healthcare professionals must deploy this technology judiciously, recognizing that responsible use is critical to achieving positive outcomes while avoiding adverse effects. Generative AI performs optimally in environments characterized by high repetition and low risk, where its reliance on historical data patterns can prove advantageous.
As we delve into the generative AI challenges for healthcare in this blog, evaluating its suitability across various healthcare activities is essential. By understanding the strengths and limitations of this technology, we can harness its potential while exercising caution and upholding the highest standards of patient care.
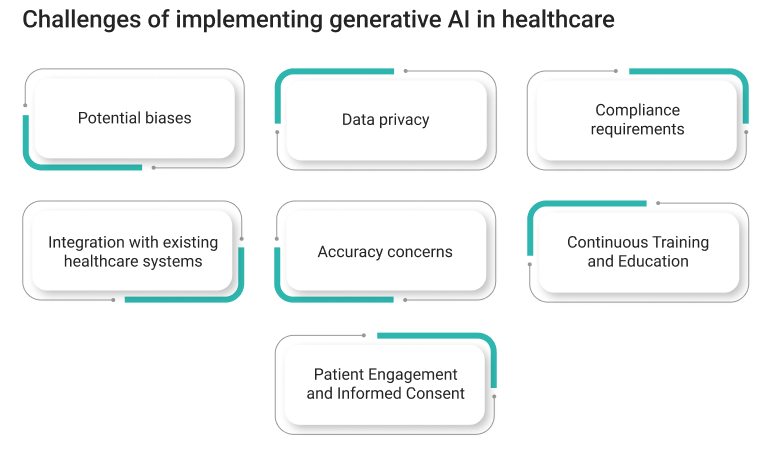
Challenges of implementing generative AI in healthcare
Let’s dive into the challenges of implementing generative AI in healthcare:
Potential biases:
It is important to be aware that implementing generative AI in healthcare can result in biased outcomes if the training data is not diverse and representative of different demographics. This bias can lead to inaccurate healthcare decisions, disproportionately affecting underrepresented groups. Generative AI models can suffer from hallucination bias, potentially resulting in harmful medical advice. To address these challenges, it’s crucial to gather training data from diverse sources and patient populations. Generative AI models must effectively address the challenge of potential bias in AI systems by sourcing diverse data, preprocessing it for balance and representation, and detecting biases within AI algorithms.
Data privacy:
Patient privacy and data security are of utmost importance in the healthcare industry. Generative AI systems require large amounts of data to function effectively, which can raise concerns about data privacy and the potential for unauthorized access or misuse. In 2019, a major healthcare provider experienced a data breach that exposed the personal information of nearly 20,000 patients. If an AI system was in place at the time, the sensitive patient data used to train the AI could have been compromised, raising concerns about data privacy and security. To address such challenges, robust data encryption, anonymization techniques, and strict access controls should be implemented to protect sensitive patient information.
Compliance requirements:
Healthcare organizations must adhere to strict regulatory standards, such as HIPAA in the United States and GDPR in the European Union. Implementing generative AI in healthcare requires ensuring that the AI systems comply with these regulations, which can be complex and time-consuming. For example, in 2016, a healthcare organization was fined $5.5 million for violating HIPAA regulations. The violation occurred when the organization failed to adequately secure a database containing sensitive patient information, which unauthorized individuals accessed. Implementing generative AI in such an environment would require strict compliance with regulatory standards. Collaboration with legal experts and regulatory bodies is essential to ensure compliance and avoid potential penalties.
Integration with existing healthcare systems:
Integrating generative AI into existing healthcare systems is challenging due to the diverse technologies and data formats used across the industry. The lack of standardization, interoperability issues between systems, and reliance on legacy infrastructures in healthcare organizations can pose significant hurdles in seamlessly integrating AI capabilities and accommodating the latest technologies. To ensure seamless integration, generative AI solutions must follow a meticulous approach involving careful planning, extensive testing for compatibility issues, and close collaboration with healthcare professionals. This collaboration should aim to understand each system’s requirements, mitigate disruptions, streamline workflow integration, and facilitate successful AI adoption within healthcare ecosystems.
Accuracy concerns:
The accuracy of AI-generated information is critical in healthcare, where incorrect diagnoses or treatment recommendations can have serious consequences. In 2018, an AI-based diagnostic tool for breast cancer screening reported a false positive rate of 9.4%, compared to the human-only rate of 8.1%. This higher rate of false positives could lead to unnecessary further testing and patient anxiety, underscoring the need for precise AI-driven healthcare solutions. Ensuring the accuracy of generative AI systems requires rigorous testing, validation, and continuous monitoring to detect and correct any errors. Additionally, it is crucial to establish clear guidelines for human oversight and intervention when necessary.
Continuous Training and Education:
As generative AI technology evolves, healthcare professionals must have the knowledge and skills to use and manage these systems effectively. This requires ongoing training and education programs to ensure that healthcare providers are up-to-date with the latest advancements in AI technology and can make informed decisions about its implementation. A survey conducted in 2019 revealed that only 16% of physicians felt prepared to use AI in their practice. This lack of preparedness highlights the need for ongoing training and education programs to ensure that healthcare professionals can effectively use and manage AI technologies
Patient Engagement and Informed Consent:
Incorporating generative AI into healthcare processes may affect the patient-provider relationship and require new approaches to patient engagement and informed consent. Healthcare providers must communicate clearly and transparently with patients about the role of AI in their care, ensuring that patients understand the benefits and potential risks associated with AI-driven healthcare solutions. This may involve developing new patient education materials and consent forms that address the unique aspects of AI-driven care. For instance, a study found that due to a lack of awareness, patients do not understand the potential risks and benefits associated with AI-driven care. This lack of understanding can hinder informed consent and negatively impact patient engagement, emphasizing the need for clear communication and education about AI in healthcare.
Future of AI in Healthcare
AI can revolutionize healthcare by providing personalized treatment plans and enhancing diagnostic accuracy. Harnessing the power of generative AI, the healthcare industry is poised for a transformative revolution that promises personalized, proactive, and precise care. At ValueLabs, we are at the forefront of this revolution, leveraging cutting-edge technologies like our enterprise generative AI platform, AiDE®, to deliver tailored solutions that address the unique challenges faced by healthcare organizations. Our team of experts meticulously analyzes individual patient data, enabling generative AI to customize treatment plans and ensure patients receive the most effective therapies. Our team successfully migrated a healthcare provider’s on-premises relational database management system (RDBMS) to a cloud-based business intelligence (BI) solution using AiDE®. We customized the AiDE® solution to meet the client’s specific requirements, incorporating features like database write-back and date filters, integrating it into their portal. This customization streamlined access and resulted in a significant 20% cost reduction, demonstrating the solution’s adaptability and tangible business impact.
Looking ahead, the future of AI in healthcare is incredibly promising. It is poised to revolutionize patient care, operations, and research. Advancements in generative AI will bring more precise diagnostics, personalized treatments, and proactive healthcare strategies. This shift promises better patient outcomes, cost savings, and enhanced capabilities for healthcare professionals, marking a significant leap toward a more efficient and patient-centric healthcare system.