Generative AI, or GenAI, has been at the forefront of transformation across industries for businesses globally. The rapid adoption of Large Language Models (LLMs) in the Healthcare sector is a testament to their transformative potential. With a market share of 1.8 billion USD in 2023, the industry is poised for exponential growth, projected to reach a staggering 22.1 billion USD by 2032. This remarkable forecast underscores the immense value LLMs bring to healthcare, empowering medical professionals to deliver more accurate diagnoses, streamline patient care, and drive innovation.
The experts opinionate that the steep demand for generative AI in healthcare will likely encourage industry leaders to prioritize:
- Creating a solid digital base
- Training employees to make them AI-ready
- Investing in data readiness
These will enable healthcare professionals to leave administrative tasks to GenAI to focus on offering better access to end-users.
Before we delve into the topic further, if you want to understand the emergence of generative AI applications in the healthcare landscape, click here. Talk to our experts today to better understand how to keep up as artificial language and machine learning algorithms continue to evolve.
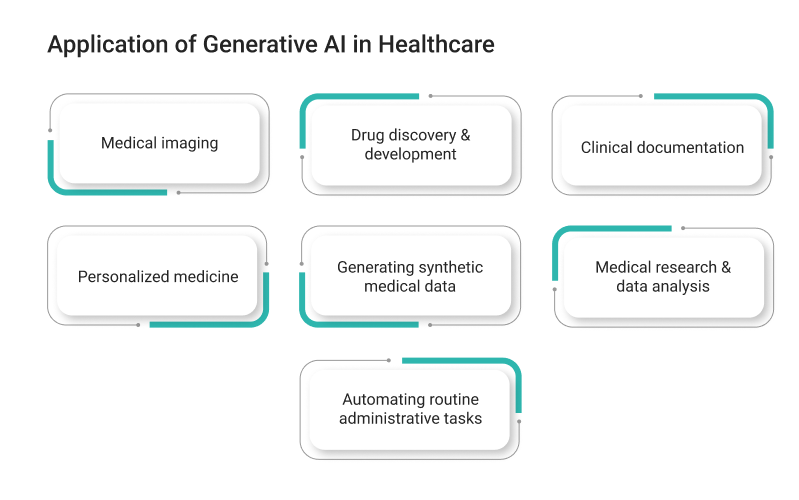
Application of Generative AI in Healthcare
The application of generative AI in the healthcare industry is widespread, and we will discuss it in depth in this section.
Medical imaging
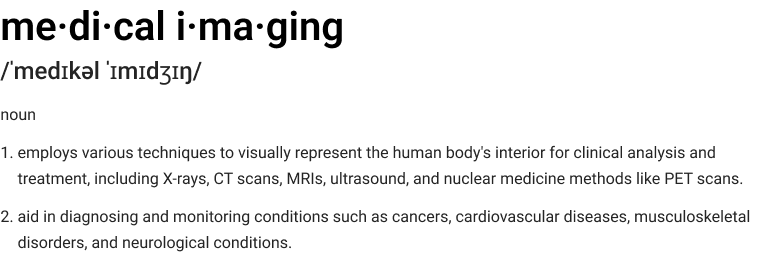
Medical imaging is a primary example of an AI application in healthcare since it detects abnormalities, guides treatment plans, and assesses therapy effectiveness. Generative AI significantly impacts medical imaging, specifically deep learning, and computer vision algorithms. The implications include image acquisition, reconstruction, enhancement, segmentation, and analysis. Trained on extensive medical image datasets, GenAI can help radiologists and healthcare professionals detect and characterize lesions, quantify tissue volumes, and predict disease progression or treatment outcomes. Generative AI models, like GANs and VAEs, optimize medical imaging processes and enhance image quality.
Denoising & reconstruction: Remove noise and artifacts from low-quality or undersampled medical images, effectively increasing their diagnostic value while reducing radiation exposure or scan times.
Data augmentation: Create synthetic medical images that resemble accurate data. This can be particularly useful when training AI models on limited datasets, helping to improve their robustness and generalization capabilities.
Image translation: Convert between different imaging modalities (e.g., MRI scans to CT scans or vice versa) to facilitate modal analysis and potentially reduce the need for multiple scans, leading to cost savings and improved patient comfort.
Drug discovery & development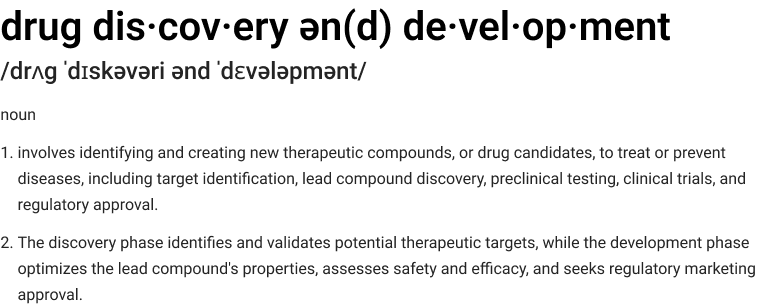
Machine learning and deep learning can potentially transform drug discovery and development by accelerating and optimizing various stages. The use of generative AI in healthcare includes aiding in target identification and validation by analyzing large datasets, leading compound discovery through virtual screening and iterative 3D drug design, and predicting pharmacokinetic and toxicological properties. These potentially reduce experimental testing and shorten development timelines.
Streamline process: Optimize drug discovery and development. Train your AI models on large datasets of existing drug molecules and their properties to learn chemical structures’ underlying patterns and rules and their associated biological activities.
Accelerate lead identification: Design and generate novel drug-like molecules with desired properties, such as increased potency, selectivity, or improved pharmacokinetic profiles. Provide a vast pool of promising candidate molecules for further evaluation to accelerate the lead identification and optimization stages.
Optimize drug candidates: Streamline existing drug candidates by generating potentially improved analogs with modified physicochemical properties or reduced off-target effects.
Data augmentation: Create synthetic data to supplement limited experimental datasets, thus improving AI models’ robustness and generalization capabilities in various drug discovery and development stages.
Clinical documentation
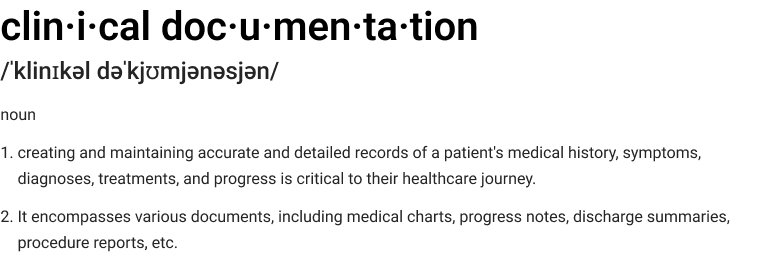
The application of generative AI in clinical documentation enables healthcare providers to deliver coordinated and consistent treatment, adhere to legal requirements, and share information efficiently for seamless collaboration.
Automated documentation generation: Generate high-quality, contextually relevant notes, summaries, or reports based on input data to reduce the time and effort required for manual documentation significantly.
Natural language generation: Convert structured data (e.g., lab values, medication lists) into natural language descriptions, making clinical documentation more readable and accessible for healthcare professionals and patients.
Translation and localization: Translate clinical documentation into different languages or adapt it to local dialects and terminologies, improving accessibility and communication across diverse healthcare settings.
Personalized medicine
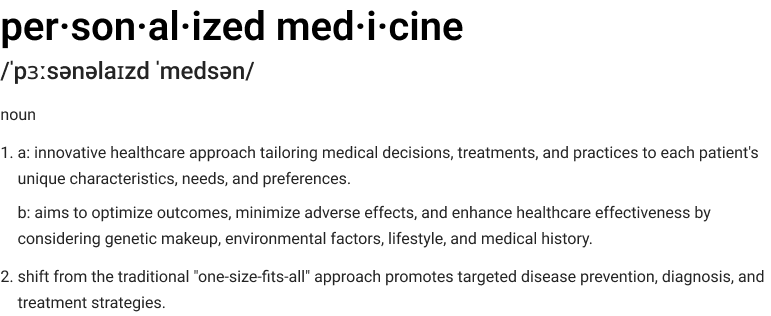
Generative AI in healthcare, especially personalized medicine, is pivotal because it leverages diverse data sources, identifies patterns, and provides tailored recommendations. It facilitates genomic analysis, predictive modeling, drug discovery, clinical decision support, and biomarker discovery, ultimately enabling early disease detection, tailored treatments, and improved patient outcomes.
Virtual patient simulation: Create virtual patient populations with diverse genetic and phenotypic characteristics. This enables researchers and clinicians to simulate and test personalized treatment strategies in silico before applying them in real-world settings.
Biomarker generation: Discover and generate novel biomarkers by analyzing multi-omics data and identifying patterns or features associated with specific conditions or treatment responses.
Patient education and engagement: Design personalized educational materials, visualizations, or interactive experiences. It helps patients better understand their conditions, treatment options, and the rationale behind customized medicine approaches.
Generating synthetic medical data
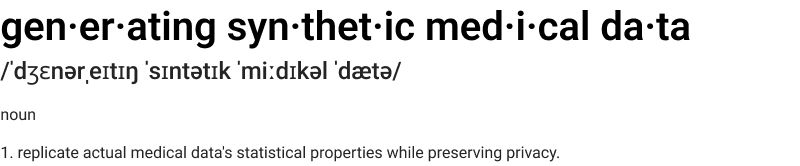
Another significant impact of generative AI in the healthcare industry involves creating artificial patient data using computational models and techniques to replicate actual medical data’s statistical properties while preserving privacy. This data can encompass electronic health records (EHRs), medical imaging, genomic data, and clinical notes generated through algorithms learning from real-world sources and capturing the original data’s underlying patterns.
High-fidelity data generation: Advanced generative AI models, such as diffusion models or large language models fine-tuned for medical data generation, can create synthetic data samples with high fidelity and realism, capturing complex relationships and nuances present in real medical data.
Multimodal data synthesis: Generate synthetic data across multiple modalities, such as integrating medical images, clinical notes, and structured EHR data, enabling comprehensive and holistic data synthesis.
Conditional generation: Conditioned on specific attributes or constraints, LLMs can generate synthetic data tailored to demographics, disease conditions, or treatment scenarios, enabling more targeted and focused analyses.
Continuous learning and adaptation: Continuously updated and fine-tuned as new real-world data becomes available, ensuring adaptation of synthetic data generation process to evolving data distributions and patterns.
Evaluation and quality control: Combined with discriminative AI models for quality control and evaluation, LLMs ensure that the generated synthetic data meets desired statistical properties and does not exhibit recognizable artifacts or biases.
Medical research & data analysis
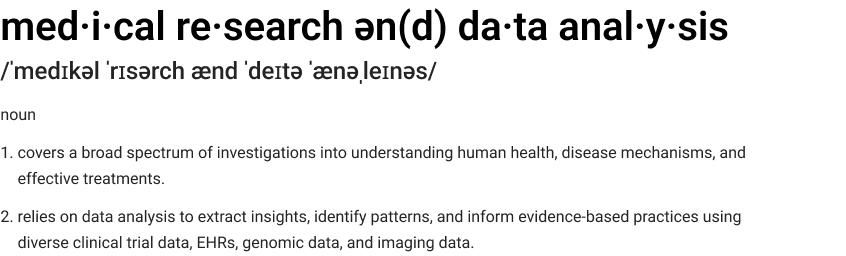
The use of generative AI in healthcare is evident as machine learning and deep learning techniques impact biomedical data integration, clinical trial optimization, predictive modeling, biomarker discovery, and literature analysis, all of which contribute to improved healthcare outcomes and efficiency.
Hypothesis generation and experiment design: Assist researchers in generating novel hypotheses, identifying promising research directions, and optimizing experiment designs based on existing knowledge and data.
Biomedical literature generation: Generate high-quality biomedical literature, such as research papers, reports, or educational materials, to facilitate the dissemination of knowledge and accelerate scientific communication.
Automating routine administrative tasks
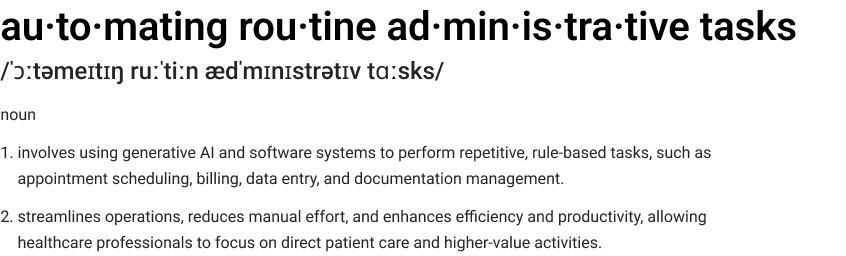
AI significantly automates healthcare administrative tasks through techniques like Natural Language Processing (NLP), Robotic Process Automation (RPA), intelligent virtual assistant, Intelligent Document Processing (IDP), and predictive analytics. By leveraging these GenAI techniques, healthcare organizations can optimize administrative processes, improve staff productivity, and enhance patient care.
Natural language generation: Generate human-like text, such as medical reports, summaries, or correspondence, reducing the need for manual document creation and improving consistency and accuracy.
Intelligent virtual assistants: Power conversational interfaces and virtual assistants, enabling more natural and contextual interactions with patients or staff, improving the user experience, and increasing the efficiency of routine administrative tasks.
Personalized communication: Generate personalized and tailored communication materials, such as appointment reminders, patient education resources, or follow-up instructions, enhancing patient engagement and adherence.
Automated documentation: Creating comprehensive and accurate medical documentation, such as encounter notes, discharge summaries, or procedure reports, reduces the administrative burden on healthcare professionals.
Generative AI Solutions in Healthcare
The real-world applications of generative AI in the healthcare industry are diverse. However, integrating generative AI models into workflows can get tricky when you factor in challenges in implementing the discussed applications in real-world scenarios.
While the prospect of GenAI deployment is promising, it requires seamless collaboration between healthcare professionals, regulatory bodies, and generative AI experts to ensure patient privacy, data integrity & privacy, and ethical use.
That’s where ValueLabs and our AiDE®-powered engineers come in. Leverage our expertise to prepare for a future of AI-augmented, efficient, and personalized healthcare solutions. Contact us today to explore the implementation of generative AI in the healthcare industry.